Effect of Behavioral Cloning on Policy Gradient Methods
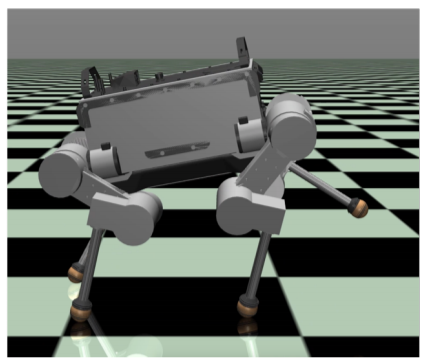
With the increasing complexity of robots and the environments they interact with, crafting manual locomotion controllers becomes a challenging task to achieve. As evident from the recent research in this field, reinforcement learning (RL) contin- ues to emerge as an attractive solution for learning locomotion policies in high- dimensional continuous domains. However, despite the impressive results of RL so far, its methods suffer from sample inefficiency and sensitivity to hyper-parameters; including but not limited to reward functions and policy initialization. Therefore, new approaches are needed to decrease the effort spent on reward engineering, which is crucial in the success of the aforementioned methods. Drawing inspiration from nature, it is often easier for an agent to learn a desired behaviour from a teacher demonstrating it rather than attempt to learn it from scratch; a process called imitation learning. This thesis explores the possibilities of warming up a policy using behavioural cloning (BC) - a type of imitation learning - to avoid getting stuck in a poor suboptimal minima and to decrease the effort spent on reward engineering. In this work we compare training an RL agent using policy gradient methods starting from a randomly initialized policy as opposed to initializing the policy through BC. This comparison is done in simulation using ANYmal robot, a sophisticated medium-dog-sized quadrupedal system. The results show that initializing a policy through BC enables capturing some of the desired behaviour features early-on without explicitly defining them into the RL problem formulation. We furthermore show that using BC for warming up produces a more robust policy - during training - towards modifications in reward functions as well as observation space, which together they form the cost manifold that policy gradient methods optimize.