Online Adaptation using Graph Networks
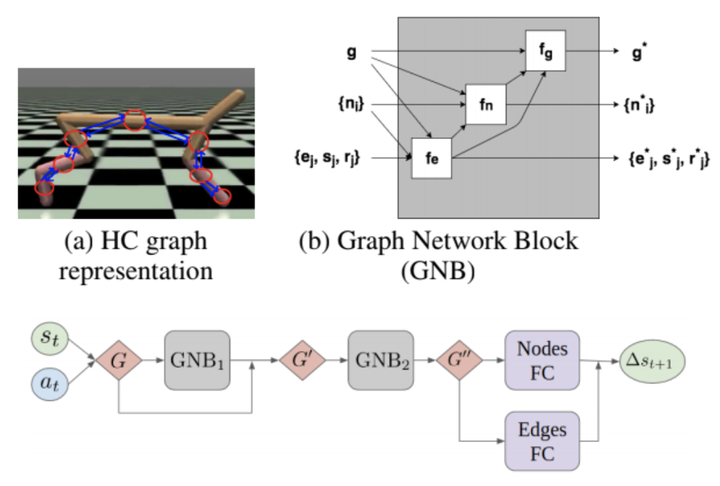
Despite the recent success of deep reinforcement learning (RL), current RL algorithms are typically unable to generalize well to unseen environment changes such as crippling or external perturbations. We hypothesize that this is due to the absence of inductive biases in these algorithms that would help transfer knowledge across tasks. In this paper, we investigate the application of graph-based relational networks to overcome this limitation. Specifically, we use a graph neural network (GNN) to learn a forward model of the environment. We use the learned model in conjunction with a model-based controller, and compare our approach against existing model-free and model-based approaches. Further, we provide insights into whether our approach helps improve generalization to new environments or not.