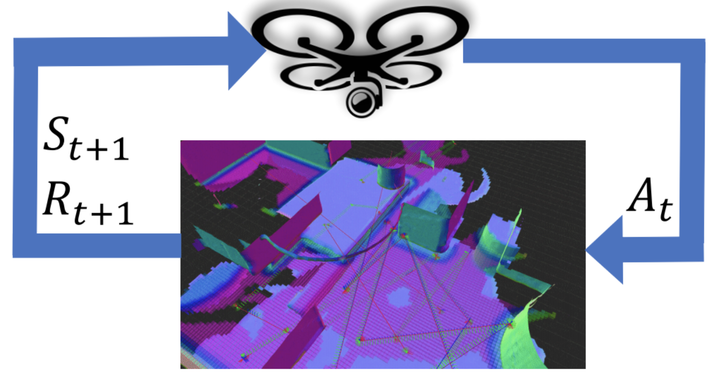
In order to enable robots to explore and assist in complex unknown environments, we propose a learning-based approach for exploring unknown cluttered environments. The underlying exploration model is an end to end neural network policy trained using reinforcement learning (RL) to use the euclidean signed distance fields (ESDFs) local map to efficiently navigate and explore the surrounding environment with no prior information about the environment itself. We evaluate our approach using different RL algorithms and reward functions. We show in simulation that the proposed exploration approach can effectively determine appropiate frontier locations to navigate to while being robust to different environment layouts. We also show that compressing the ESDF local map to a meaningful descriptor accelarates the training process.